Transforming Agriculture with Machine Learning: Unlocking Potential through Datasets
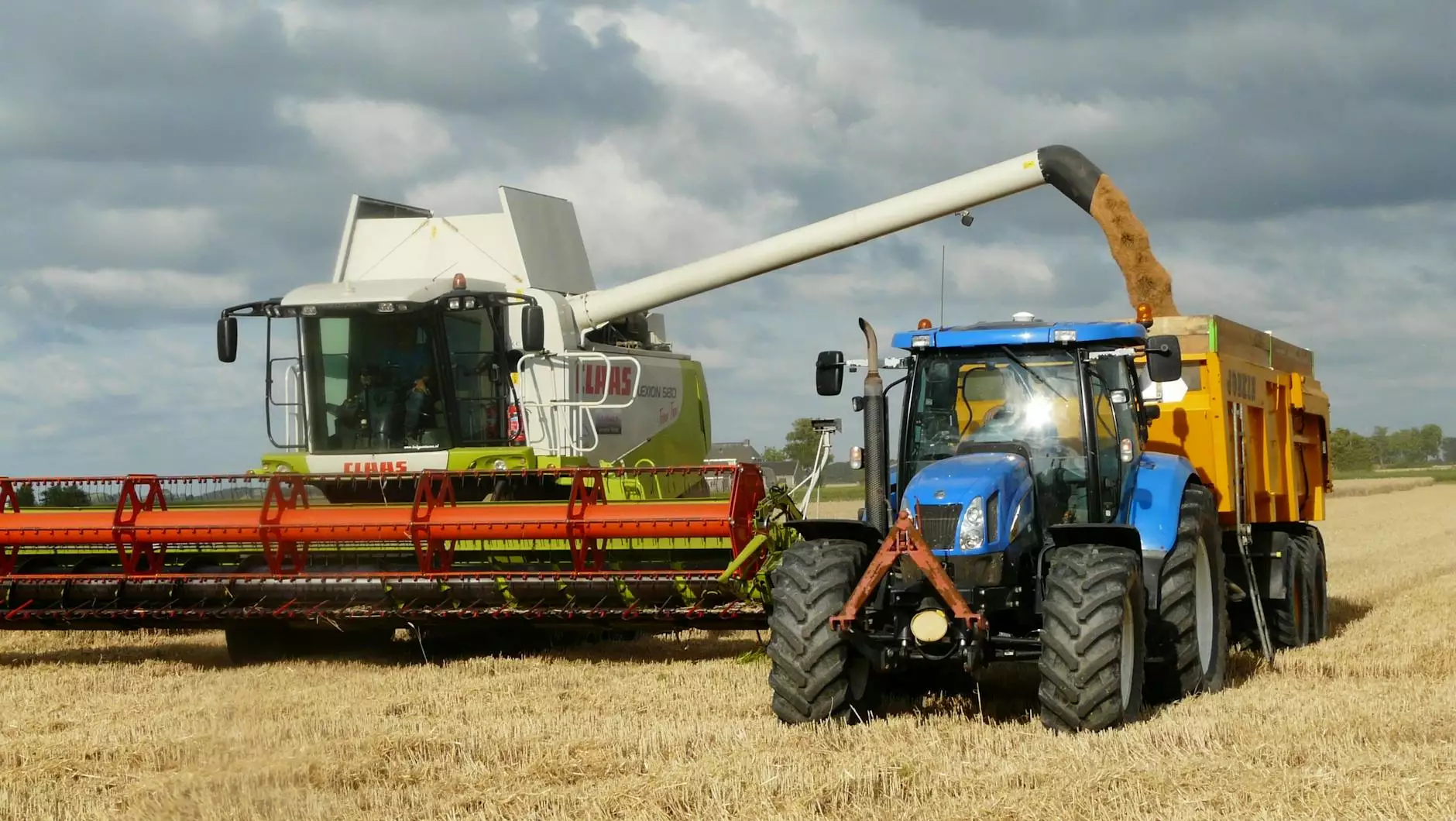
In today's fast-paced world, the intersection of technology and agriculture has become increasingly advantageous. This article delves deep into how agriculture datasets for machine learning are being utilized to propel business growth in the farming sector.
The Power of Data in Agriculture
Data has played a crucial role in the evolution of agricultural practices. With the advent of precision agriculture and the growing demand for sustainable practices, leveraging data has become essential in:
- Improving crop yields
- Reducing waste
- Enhancing soil management
- Optimizing resource use
Through robust data analysis, farmers can make informed decisions that not only increase profitability but also promote environmental sustainability. This is where agriculture datasets for machine learning become invaluable tools.
What are Agriculture Datasets?
Agriculture datasets are collections of information relevant to farming practices. These datasets can include:
- Climate data: Temperature, rainfall, humidity, and other weather-related factors.
- Soil data: pH levels, nutrient content, moisture levels, and type of soil.
- Crop yield data: Historical yield reports, harvest times, and disease prevalence.
- Pest and disease data: Incidence of pests and specific crop diseases.
- Market data: Pricing trends and demand forecasts.
When combined, these datasets create a comprehensive view that can inform machine learning models.
Machine Learning in Agriculture: A Game Changer
Machine learning (ML) is a branch of artificial intelligence that allows systems to learn from data and improve over time without being explicitly programmed. In agriculture, ML algorithms analyze data to derive insights that can enhance decision-making. Here's how:
1. Predictive Analytics
By utilizing agriculture datasets for machine learning, farmers can employ predictive analytics to forecast various outcomes, including crop yields and pest invasions. For instance, analyzing historical yield data in conjunction with weather patterns allows for:
- Better Planning: Farmers can plan planting seasons better, ensuring that they minimize risks associated with climate variability.
- Resource Allocation: Efficient distribution of resources, such as water and fertilizers, becomes feasible through predictive forecasting.
2. Precision Agriculture
Machine learning enables precision agriculture, where farmers can optimize farming practices by:
- Monitoring Crop Health: Drones equipped with sensors can capture real-time data that algorithms analyze for crop health assessments.
- Automating Irrigation Systems: Intelligent irrigation systems adjust water supply based on data analytics, conserving water resources.
Business Applications: A Case Study Approach
Numerous businesses are efficiently implementing machine learning in agriculture, each utilizing agriculture datasets for machine learning differently to overcome challenges and gain a competitive edge:
Case Study 1: Smart Agriculture Technologies
Smart Agriculture Technologies provides IoT solutions to farmers. Utilizing detailed datasets, they help farmers monitor soil health:
- Soil Sensors: Collect data on moisture and nutrient levels.
- Data Analysis: The company’s algorithms predict optimal planting times, which helps to boost yields significantly.
Case Study 2: Crop Monitoring Platforms
Crop monitoring platforms utilize satellite imagery and machine learning:
- Image Recognition: The technology identifies diseased plants quickly.
- Timely Alerts: Farmers receive alerts and recommendations to treat affected areas, enhancing overall productivity.
The Future of Agriculture and Machine Learning
As technology continues to evolve, the role of machine learning in agriculture is only set to increase. The future holds several exciting prospects:
1. Comprehensive Data Integration
Future agriculture will rely heavily on integrating various datasets—from climate to market trends—leading to:
- Holistic Understanding: Farmers will comprehend the complete ecosystem affecting their crops.
- Enhanced Sustainability: Agri-tech companies will develop solutions that promote sustainability while maximizing output.
2. Autonomous Farming
With the rise of autonomous systems, we may soon witness:
- Self-driving Tractors: Machines that will autonomously navigate fields, optimizing fuel and fertilizers' usage.
- Automated Pest Control: Drones may be utilized for targeted pest control, minimizing chemical usage and environmental impact.
Challenges and Considerations
While the benefits of agriculture datasets for machine learning are profound, some challenges exist:
1. Data Privacy and Security
Farmers must be cautious about sharing data, ensuring that their competitive edge remains safeguarded.
2. Technical Limitations
The implementation of these technologies requires significant investment, which may not be feasible for all, particularly smallholder farmers.
3. Need for Training
As technology integrates into agriculture, there is a necessitation for education and training to ensure farmers can utilize these tools effectively.
Conclusion: A Collaborative Future in Agriculture
In conclusion, the agriculture dataset for machine learning represents an enormous opportunity to transform the agricultural sector. By harnessing this data effectively, businesses can drive innovation, enhance productivity, and support sustainable practices. As companies like Keymakr prioritize innovative solutions in Home Services, Keys & Locksmiths, the agriculture sector must embrace machine learning as a cornerstone for growth and resilience.
As we move forward into a future that embraces technology, the synergy between agriculture and machine learning will undoubtedly yield a harvest of success, bridging traditional practices with modern advancements.