Mastering Data Labeling: A Comprehensive Guide for Businesses
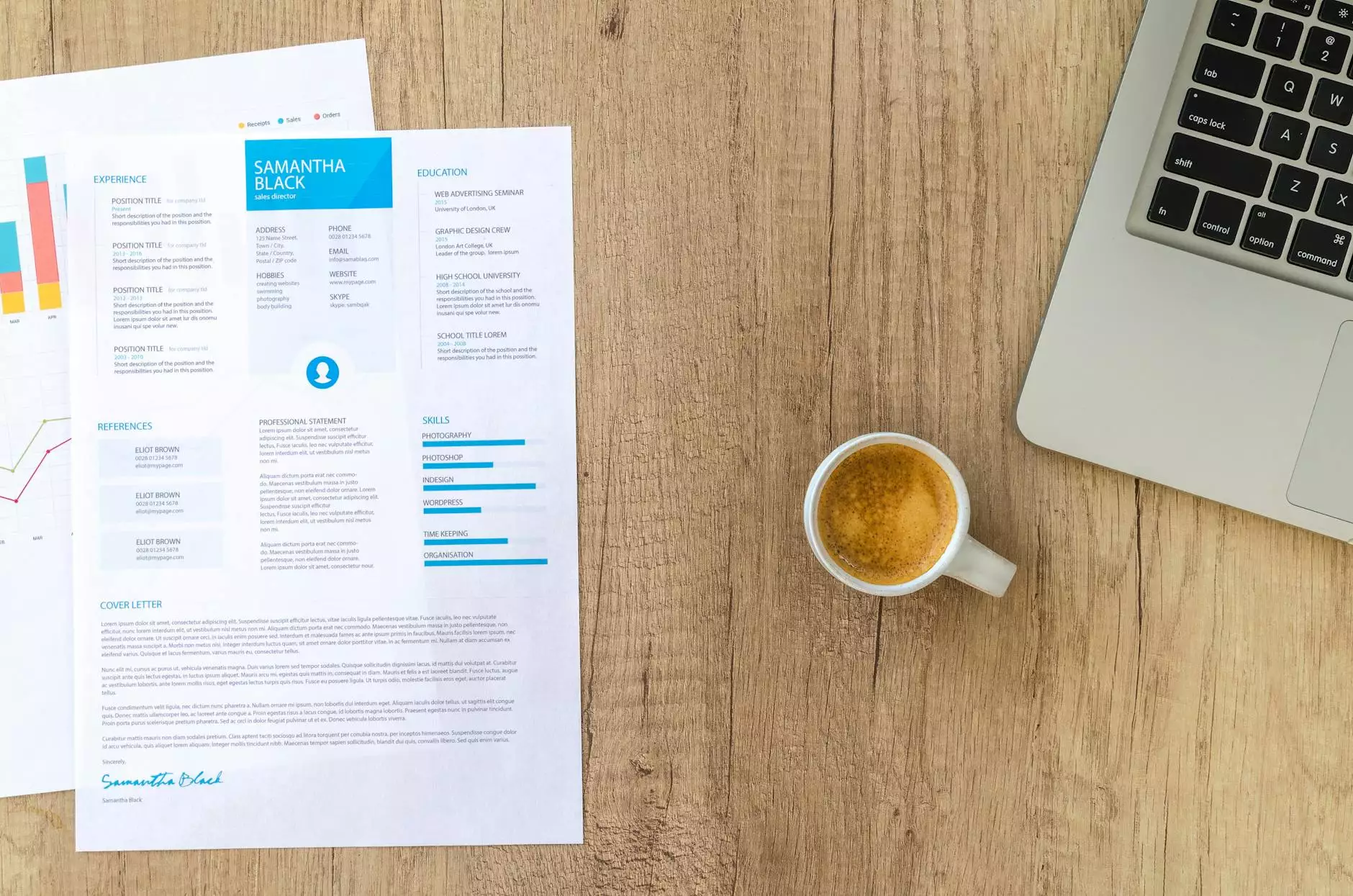
The digital era has transformed the way businesses operate, placing data at the forefront of decision-making processes. Among the myriad aspects of data handling, one critical element stands out: data labeling. It serves as a linchpin in the world of artificial intelligence and machine learning, impacting how well businesses can utilize data for growth and innovation. In this article, we delve deep into the concept of data labeling, its significance, the tools and platforms available, and how Keylabs AI is expertly positioned to assist businesses in navigating this intricate landscape.
Understanding Data Labeling
Data labeling is the process of annotating or tagging raw data to make it understandable for machine learning models. It involves affixing specific labels to data inputs, such as images, text, audio, or video, enabling algorithms to learn from these labeled datasets. Essentially, it's the foundation upon which supervised machine learning models are built, allowing them to predict outputs based on new, unlabeled data.
The Importance of Data Labeling
In the realm of AI, the quality and quantity of data directly influence model accuracy. Here’s why data labeling is paramount for businesses:
- Improved Accuracy: Properly labeled data ensures that machine learning models can accurately interpret and predict outcomes.
- Enhanced Learning: Labeled datasets allow models to learn nuances in data patterns and correlations, leading to better performance in real-world applications.
- Faster Development: High-quality labeled data accelerates the training process, enabling quicker deployment of AI solutions.
- Better Decision Making: With accurate predictions, businesses can make informed decisions that drive success and innovation.
Applications of Data Labeling in Business
Data labeling is ubiquitous across various industries, driving numerous applications that boost efficiency, accuracy, and productivity. Below are some key areas where data labeling plays an essential role:
1. Image Recognition
In sectors like retail and healthcare, image recognition is crucial. Labeled images help train models for applications such as:
- Facial recognition: Used for security and identification purposes.
- Inventory management: Automating the identification of products.
- Medical imaging: Aiding in the detection of diseases from scans.
2. Natural Language Processing (NLP)
As businesses cultivate stronger connections with customers, NLP facilitates communication through chatbots and virtual assistants. Data labeling helps in:
- Sentiment analysis: Understanding customer feedback and opinions.
- Text classification: Categorizing emails or documents for better information retrieval.
3. Autonomous Vehicles
In the automotive industry, data labeling is integral for training self-driving cars. Key applications include:
- Object detection: Recognizing pedestrians, traffic signs, and other vehicles.
- Path planning: Enabling vehicles to understand navigational challenges.
Choosing the Right Data Labeling Tool
With the growing demand for high-quality data labeling, businesses need the right tools to streamline these processes. Here are some considerations when selecting a data annotation tool:
1. Scalability
As project requirements grow, your data labeling tool should be able to accommodate increased volumes of data without compromising on quality. Look for platforms that can handle large datasets efficiently.
2. Flexibility
Different projects may require different types of data labeling. Choose a tool that supports multiple annotation types, including bounding boxes, polygons, and semantic segmentation.
3. Collaboration Features
Data labeling often involves teams of annotators. A good tool should facilitate collaboration, allowing multiple team members to work on the same project simultaneously, track changes, and share feedback.
4. Quality Control
Ensure that the tool you choose incorporates quality assurance mechanisms such as review modes and accuracy assessments, minimizing the margin for error.
Why Keylabs AI Stands Out
At Keylabs AI, we recognize the critical role that data labeling plays in the success of AI initiatives. Our data annotation platform is specifically designed to meet the diverse needs of businesses, offering:
1. Advanced Annotation Tools
We provide a suite of intuitive tools that allow for precise and flexible data labeling, ensuring all types of inputs—from images to text—are accurately annotated to meet your project’s goals.
2. Expert Human Labelers
While automation is powerful, the human touch is irreplaceable. Our team of expert labelers ensures that every project benefits from a meticulous, human-centered approach, enhancing the quality of the labeled data.
3. Enhanced Security
Data privacy is crucial. Keylabs AI implements stringent security protocols to ensure your data is protected throughout the labeling process, providing peace of mind to our clients.
4. Comprehensive Support
From setup to execution, our dedicated support team assists clients every step of the way, ensuring that your data labeling projects are seamless and efficient.
Best Practices for Data Labeling
To maximize the effectiveness of your data labeling efforts, consider the following best practices:
1. Define Clear Guidelines
Establish precise labeling standards before initiating any project. Clear guidelines ensure consistency, reduce discrepancies, and improve inter-annotator agreement.
2. Invest in Training
Whether utilizing in-house teams or third-party services, invest time in training your annotators. Well-trained personnel are more capable of producing high-quality results that meet your business needs.
3. Regular Quality Checks
Implement routine quality checks throughout the labeling process to maintain high standards. This can involve random audits or setting up a system for feedback and corrections.
4. Encourage Feedback
Solicit feedback from your annotators regarding the process. Continuous improvement is essential; input from your team can reveal pain points and areas for enhancement.
Future Trends in Data Labeling
As businesses embrace AI, several trends in data labeling are emerging that will shape how organizations approach this critical task:
1. Automated Labeling
While human annotation is invaluable, automated labeling techniques—utilizing algorithms to pre-label data—are on the rise. These tools can save time and reduce costs, although they still require human oversight to ensure accuracy.
2. Collaborative Annotation Platforms
The future of data labeling lies in collaborative platforms that enable real-time contributions and feedback from multiple users. Enhanced collaboration can lead to improved quality and quicker turnaround times.
3. Mixed Reality Data Annotation
As mixed reality technologies advance, expect to see innovative data labeling techniques that leverage augmented and virtual reality to label complex datasets more intuitively.
Conclusion
In conclusion, data labeling is an indispensable element of the AI landscape that businesses cannot afford to overlook. By investing in quality data labeling, organizations can significantly enhance their machine learning efforts, ultimately leading to better decision-making, improved products, and increased competitiveness in the marketplace. With Keylabs AI’s innovative data annotation platform and dedicated support, businesses can navigate the complexities of data labeling with confidence and achieve their desired outcomes more effectively. Embrace the power of labeled data today and watch your business thrive in the digital age.